Human ethical standards aren’t adequate for artificial intelligence
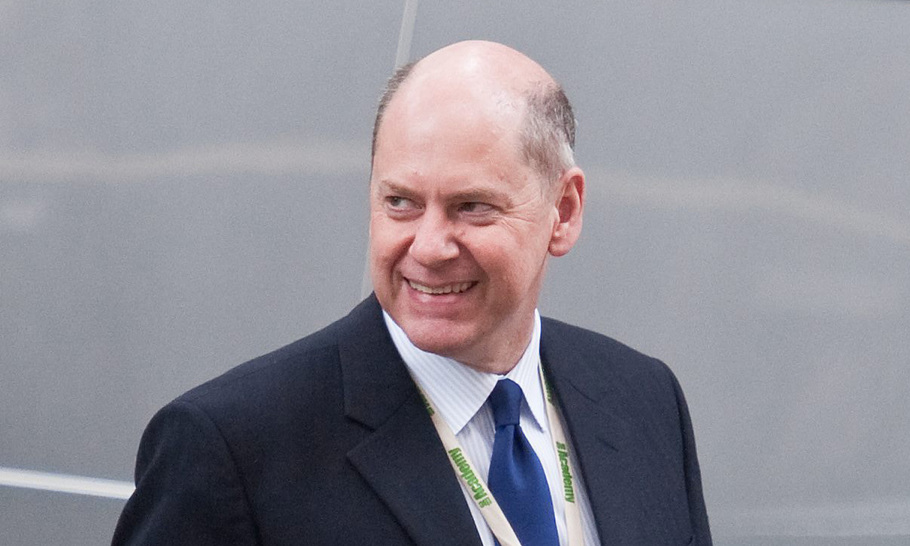
Jonathan Evans (MaxPPP)
Jonathan Evans’s work at the Committee on Standards into Public Life has taken a necessary first step towards the examination of the impact of artificial intelligence (AI) on public sector ethics. His account of this work for TheArticle elucidates the official approach with clarity and insight.
However, approaching the ethics of AI via the Nolan principles occludes a fundamental point. These are principles designed to be imposed on human decision-makers. Regulating AI’s human masters may be necessary, but it is not sufficient on its own. It is far less clear that standards designed for humans have the philosophical scope to cover decision-making augmented by AI.
Indeed the whole point of embracing AI for greater efficiency is that AI creates an often inscrutable process of decision making, a so-called “black box”, that works precisely because it does not exactly mirror human cognition. Can we simply apply the same ethical standards when thinking about a different form of computational cognition?
Based on current technology there are essentially five types of machine learning algorithms that drive AI. Each has a different underlying logic and philosophy. All of the approaches share three parts: expression, assessment and optimisation. In theory, machines have the capacity to self-optimise to improve their learning capability — perhaps infinitely. However, all the data, method and principles used for assessment are determined by humans. So while, at least in theory, it is impossible for current forms of AI to replace humans, it is entirely possible for them to become too complicated for humans to understand them.
Evans is quite right to call for better disclosure, but the point is that often, when it comes to AI, this is just not possible. Data scientists often have to expend forensic effort to understand how the AI algorithms are working. This challenge will increase exponentially over the next ten years when quantum computing appears and the speed of machine learning will very likely eclipse our capabilities.
In this sense, the Committee on Standards in Public Life is approaching the regulation of AI in the wrong way. Legal and regulatory frameworks such as the Nolan Principles, which Lord Evans cites, typically operate around a clear sense of who is acting, what their mindset was at the time of the action and where the act took place. These principles are fine for regulating public sector employees, but a distinction in regulatory practice has to be drawn between the operator and the technology. They are no longer synonymous, as in the case of a civil servant using or misusing a PC. We only have to think of various AI scenarios (admittedly not all within the public sector) to see where the logic breaks down. Who is responsible in the case of a crash involving AI vehicles? At a fundamental level, how does existing regulation apply when agency over regulated activity is taken away from humans?
To take the example of surveillance, the Nolan principles only deal with a tiny fraction of the issues involved. AI’s capacity to scale up is a separate threat from its human masters. It replaces the human who watch the feeds and becomes a different type of system altogether, turning CCTV from passive into active observation. As the Chinese experience of a “total surveillance state” illustrates, this a problem even with current levels of technological sophistication.
Equally, the challenge AI presents is also to do with aggregated data. We only have to imagine the temptation to aggregate data from Automatic Number Plate Recognition, mobile phone networks and CCTV for instance and then apply AI to an integrated data set. Extremely clear data boundaries need to be thought about as part of the AI regulatory framework.
The promise of AI is that it will allow machines to spot patterns from data that humans cannot, while simultaneously making decisions faster. Almost inevitably these patterns will create currently unpredictable forms of bias. If we aren’t clear about the goals we set for AI, the danger is that an unintended disparity emerges.
We simply have to think about the simplistic example of the command “get me to the train station as quickly as possible”. In order for this to be carried out acceptably we need to lay out the rules of the road, otherwise a trail of mayhem would be left behind. This becomes more complicated when we are dealing with data and problems where we simply do not know what the rules of the game are. Evans suggests that “ethical risks should be properly flagged and mitigated”, but this does not account for the type of “unknown unknowns” that AI is likely to reveal.
There is a historical precedent for how we might tackle the herculean challenge of protecting society from technological development. As the Industrial Revolution transformed the economy of the West and lives of its citizens, it soon became apparent that the marketplace rules that had worked effectively for agrarian mercantilism were no longer appropriate for industrial capitalism. Perhaps the key lesson is to look at regulating the effects of the technology, as well as the technology itself and its operators.